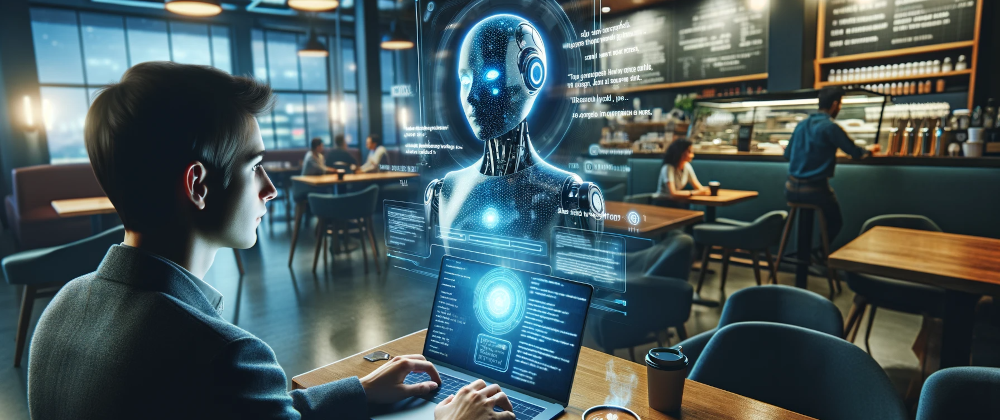
In this blog post, we'll build a Next.js chatbot that runs on your computer. We'll use Llama.cpp to serve the OpenHermes 2.5 Mistral LLM (large language model) locally, the Vercel AI SDK to handle stream forwarding and rendering, and ModelFusion to integrate Llama.cpp with the Vercel AI SDK. The chatbot will be able to generate responses to user messages in real-time.
The architecture looks like this:
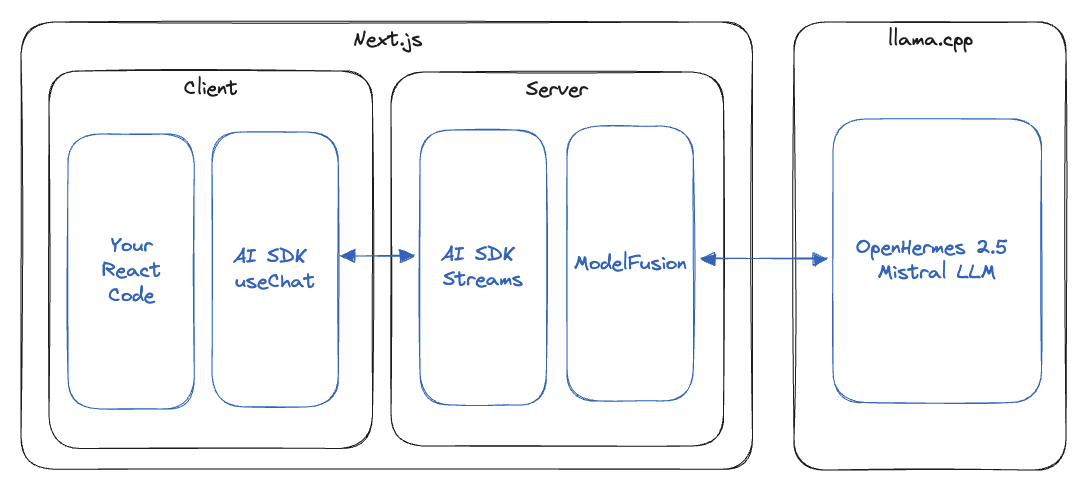
You can find a full Next.js, Vercel AI SDK, Llama.cpp & ModelFusion starter with more examples here: github/com/lgrammel/modelfusion-Llamacpp-nextjs-starter
This blog post explains step by step how to build the chatbot. Let's get started!
Setup Llama.cpp
The first step to getting started with our local chatbot is to setup Llama.cpp.
Llama.cpp is an LLM (large language model) inference engine implemented in C++ that allows us to run LLMs like OpenHermes 2.5 Mistral on your machine. This is crucial for our chatbot as it forms the backbone of its AI capabilities.
Step 1: Build Llama.cpp
Llama.cpp requires you to clone the repository and build it on your machine. Please follow the instructions on the Llama.cpp README:
-
Open your terminal or command prompt.
-
Clone the repository:
git clone https://github.com/ggerganov/llama.cpp
cd llama.cpp -
Compile llama.cpp:
- Linux/Mac: Run
make
- Windows or other setups: Please follow the instructions on the Llama.cpp README.
- Linux/Mac: Run
Step 2: Downloading OpenHermes 2.5 Mistral GGUF
Once Llama.cpp is ready, you'll need to pull the specific LLM we will be using for this project, OpenHermes 2.5 Mistral.
-
Download the OpenHermes 2.5 Mistral model from HuggingFace. I'll use
openhermes-2.5-mistral-7b.Q4_K_M.gguf
in this tutorial. -
Move the model file into the
models/
directory of your local Llama.cpp repository.
Llama.cpp runs LLMs in a format called GGUF
(GPT-Generated Unified Format). You can find many GGUF models on HuggingFace. 4-bit quantized models that fit in your machine's memory, e.g. 7B param models on a 8GB or 16GB machine, are usually the best models to run.
Quantization involves reducing the precision of the numerical values representing the model's weights, often from 32-bit floating points to lower precision formats like 4-bit. This decreases the model's memory footprint and computational requirements.
Step 3: Start the Llama.cpp Server
You can now start the Llama.cpp server by running the following command in your terminal (Mac/Linux):
./server -m models/openhermes-2.5-mistral-7b.Q4_K_M.gguf
After completing these steps, your system is running a Llama.cpp server with the OpenHermes 2.5 Mistral model, ready to be integrated into our Next.js chatbot.
Creating the Next.js Project
The next step is to create the foundational structure of our chatbot using Next.js. Next.js will be used to build our chatbot application's frontend and API routes.
Here are the steps to create the Next.js project:
-
Execute the following command in your terminal to create a new Next.js project:
npx create-next-app@latest llamacpp-nextjs-chatbot
-
You will be prompted to configure various aspects of your Next.js application. Here are the settings for our chatbot project:
Would you like to use TypeScript? Yes
Would you like to use ESLint? Yes
Would you like to use Tailwind CSS? Yes
Would you like to use `src/` directory? Yes
Would you like to use App Router? (recommended) Yes
Would you like to customize the default import alias? NoThese settings enable TypeScript for robust type-checking, ESLint for code quality, and Tailwind CSS for styling. Using the src/ directory and App Router enhances the project structure and routing capabilities.
-
Once the project is initialized, navigate to the project directory:
cd llamacpp-nextjs-chatbot
By following these steps, you have successfully created and configured your Next.js project. This forms the base of our chatbot application, where we will later integrate the AI functionalities using Llama.cpp and ModelFusion. The next part of the tutorial will guide you through installing additional libraries and setting up the backend logic for the chatbot.
You can verify your setup by running npm run dev
in your terminal and navigating to http://localhost:3000 in your browser. You should see the default Next.js page.
Installing the Required Libraries
We will use several libraries to build our chatbot. Here is an overview of the libraries we will use:
- Vercel AI SDK: The Vercel AI SDK provides React hooks for creating chats (
useChat
) as well as streams that forward AI responses to the frontend (StreamingTextResponse
). - ModelFusion: ModelFusion is a library for building multi-modal AI applications that I've been working on. It provides a
streamText
function that calls AI models and returns a streaming response. ModelFusion also contains a Llama.cpp integration that we will use to access the OpenHermes 2.5 Mistral model. - ModelFusion Vercel AI SDK Integration: The @modelfusion/vercel-ai integration provides a
ModelFusionTextStream
that adapts ModelFusion's text streaming to the Vercel AI SDK's streaming response.
You can run the following command in the chatbot project directory to install all libraries:
npm install --save ai modelfusion @modelfusion/vercel-ai
You have now installed all the libraries required for building the chatbot. The next section of the tutorial will guide you through creating an API route for handling chat interactions.
Creating an API Route for the Chatbot
Creating the API route for the Next.js app router is the next step in building our chatbot. The API route will handle the chat interactions between the user and the AI.
Create the api/chat/
directory in src/app/
directory of your project and create a new file named route.ts
to serve as our API route file.
The API route requires several important imports from the ai
, modelfusion
, and @modelfusion/vercel-ai
libraries. These imports bring in necessary classes and functions for streaming AI responses and processing chat messages.
import { ModelFusionTextStream, asChatMessages } from "@modelfusion/vercel-ai";
import { Message, StreamingTextResponse } from "ai";
import { llamacpp, streamText } from "modelfusion";
We will use the edge runtime:
export const runtime = "edge";
The route itself is a POST request that takes a list of messages as input:
export async function POST(req: Request) {
// useChat will send a JSON with a messages property:
const { messages }: { messages: Message[] } = await req.json();
// ...
}
We initialize a ModelFusion text generation model for calling the Llama.cpp chat API with the OpenHermes 2.5 Mistral model. The .withChatPrompt()
method creates an adapted model for chat prompts:
const model = llamacpp
.CompletionTextGenerator({
promptTemplate: llamacpp.prompt.ChatML, // OpenHermes uses the ChatML prompt format
temperature: 0,
cachePrompt: true, // Cache previous processing for fast responses
maxGenerationTokens: 1024, // Room for answer
})
.withChatPrompt();
Next, we create a ModelFusion chat prompt from the AI SDK messages:
const prompt = {
system: "You are an AI chatbot. Follow the user's instructions carefully.",
// map Vercel AI SDK Message to ModelFusion ChatMessage:
messages: asChatMessages(messages),
};
The asChatMessages
helper converts the messages from the Vercel AI SDK to ModelFusion chat messages.
With the prompt and the model, you can then use ModelFusion to call Llama.cpp and generate a streaming response:
const textStream = await streamText({ model, prompt });
Finally you can return the streaming text response with the Vercel AI SDK. The ModelFusionTextStream
adapts ModelFusion's streaming response to the Vercel AI SDK's streaming response:
// Return the result using the Vercel AI SDK:
return new StreamingTextResponse(ModelFusionTextStream(textStream));
Adding the Chat Interface
We need to create a dedicated chat page to bring our chatbot to life on the frontend. This page will be located at src/app/page.tsx
and will leverage the useChat hook from the Vercel AI SDK. The useChat
hook calls the /api/chat
route and processes the streaming response as an array of messages, rendering each token as it arrives.
// src/app/page.tsx
"use client";
import { useChat } from "ai/react";
export default function Chat() {
const { messages, input, handleInputChange, handleSubmit } = useChat();
return (
<div className="flex flex-col w-full max-w-md py-24 mx-auto stretch">
{messages.map((message) => (
<div
key={message.id}
className="whitespace-pre-wrap"
style={{ color: message.role === "user" ? "black" : "green" }}
>
<strong>{`${message.role}: `}</strong>
{message.content}
<br />
<br />
</div>
))}
<form onSubmit={handleSubmit}>
<input
className="fixed bottom-0 w-full max-w-md p-2 mb-8 border border-gray-300 rounded shadow-xl"
value={input}
placeholder="Say something..."
onChange={handleInputChange}
/>
</form>
</div>
);
}
It's important to clean up the global styles for a more visually appealing chat interface. By default, the Next.js page is dark. We clean up src/app/globals.css
to make it readable:
@tailwind base;
@tailwind components;
@tailwind utilities;
Running the Chatbot Application
With the chat page in place, it's time to run our chatbot app and see the result of our hard work.
You can launch the development server by running the following command in your terminal:
npm run dev
You can now navigate to http://localhost:3000 in your browser to see the chat page. You can interact with the chatbot by typing messages into the input field. The chatbot will respond to your messages in real-time.
Below is a screenshot of what you can expect your chatbot interface to look like when you run the application:
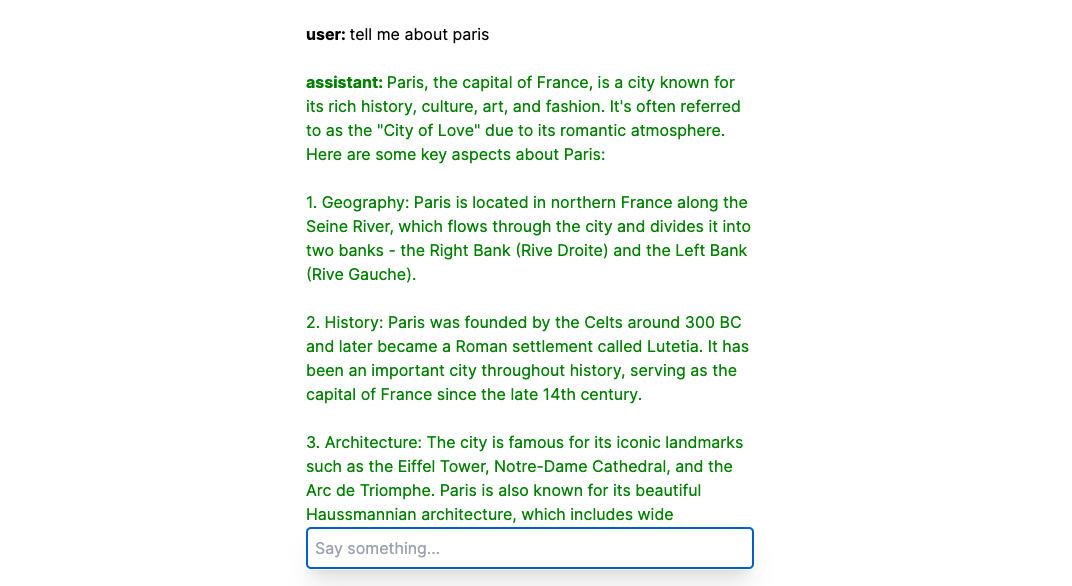
Conclusion
And there you have it—a fully functional local chatbot built with Next.js, Llama.cpp, and ModelFusion at your fingertips. We've traversed the path from setting up our development environment, integrating a robust language model, and spinning up a user-friendly chat interface.
The code is intended as a starting point for your projects. Have fun exploring!